AI-Driven Threat Detection & Response
The Problem
Efficient threat detection in cybersecurity faces critical challenges, including managing false positives and negatives, keeping pace with sophisticated and evolving attack methods, analyzing vast amounts of data in real-time, and providing actionable insights with minimal resources. Traditional detection solutions often lack the context and visibility needed to differentiate between benign and malicious activities, especially in environments with encrypted traffic, fragmented tools, and emerging technologies like IoT and cloud platforms.
Among this sea of alerts lies the critical challenge of detecting true threats—those subtle, often hidden signals that indicate malicious activity—without drowning in false positives. High-fidelity detection, the ability to pinpoint real threats with precision and accuracy, has become the holy grail for security teams. Yet, separating actionable signals from the surrounding noise remains one of the most complex and persistent problems, demanding advanced tools, innovative methodologies, and a shift in how we approach threat detection and response.
Most organizations today lack the manpower and the visibility needed to effectively detect and investigate security alerts triggered by their own solutions. The traditional approach of correlating events from different sources using SIEM is an important step in prioritizing investigations. However, trying to understand the scope of a threat through log data is rarely enough as the log data is often disparate and lacks context, requiring significant effort to normalize and correlate the logs into actionable insights.
As a result, the time it takes to respond to incidents remains a significant bottleneck, with many organizations struggling to act quickly due to the complexity of correlating data from different tools and the reliance on highly skilled analysts.
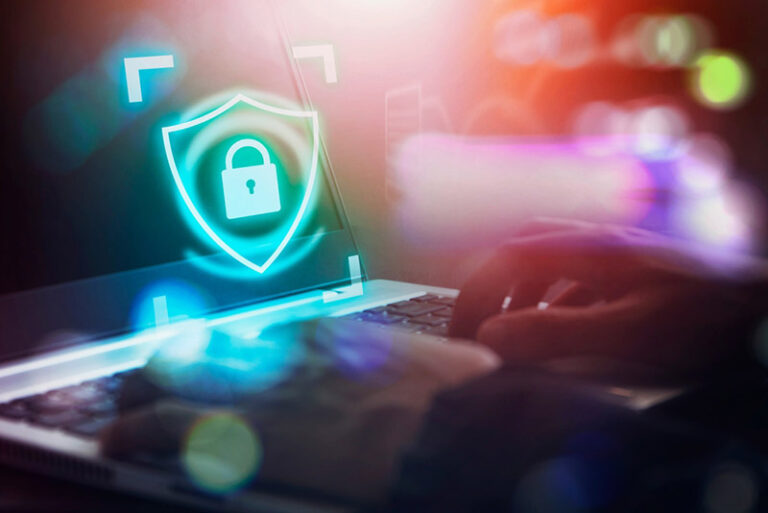
The Solution
- Real-Time AI-Powered Anomaly Detection
Detect sophisticated threats from lateral movement, insider threat or malware attacks and automate alerts faster and more efficiently, while also integrating with SIEM platforms and EDR tools to enhance organizations overall security posture. - Automated Incident Response (IR) Engine
Accelerate response workflows by automatically investigating alerts received from a SIEM or other detection tools. Shorten response times, reduce manual effort, and empower even small teams of operators to handle threats with efficiency and effectiveness. - Integration with SOC Tools and Workflows
Centralize threat data, automate repetitive tasks, and streamline incident response reducing the time and effort required to correlate alerts, identify root causes, and take action. Ultimately, minimizing the risk of oversight or delayed responses. - Make Alert Validation Easy
Remove skill set barriers so that security professionals at all levels can quickly validate threats, handle more complex investigations and escalate fewer tickets. Gain deep insight into the network, protocols, applications, and actions allowing analysts to quickly assess and respond.